6 Key Data Quality Dimensions: Insights and Practical Application
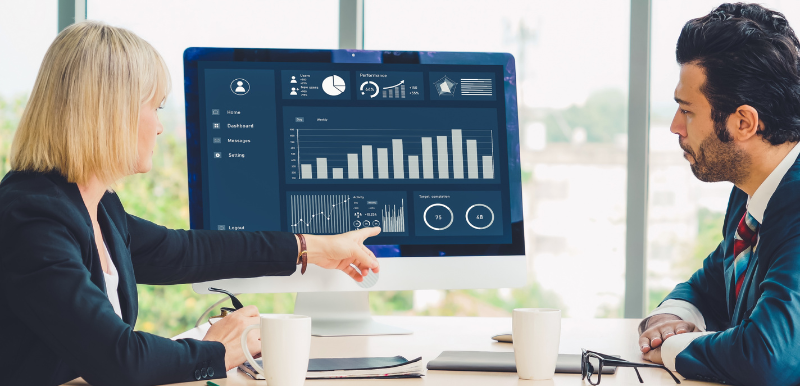
Image Source: Freepik
One of the most common challenges faced by professionals in working with key performance indicators (KPIs) relates to data. They grapple with collecting and analyzing data to establish targets accurately, as indicated by 42% of respondents in The KPI Institute’s State of Strategy Management Practice 2023 Report.
This is particularly important as the collected data is expected to be of high quality and “fit for their intended uses in operations, decision making, and planning,” according to the book “Modern Data Strategy,” by Mike Fleckenstein and Lorraine Fellows. Drawing from its advisory experience, The KPI Institute recommends employing the following data quality dimensions as a framework for assessing your data (see Figure 1).
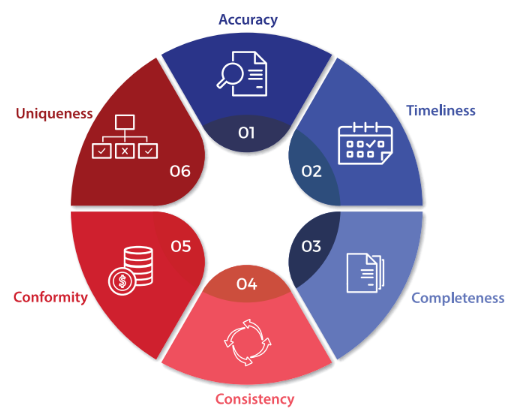
Figure 1. Data Quality Dimensions | Source: Certified KPI Professional training program
Overcoming Issues with Data Quality Dimensions
Figure 2 highlights a dataset that has encountered significant data quality issues. Through an initial audit, several faulty elements have been identified, revealing potential inaccuracies that could have an adverse impact. This section presents approaches for effectively resolving these faulty elements to improve data reliability.
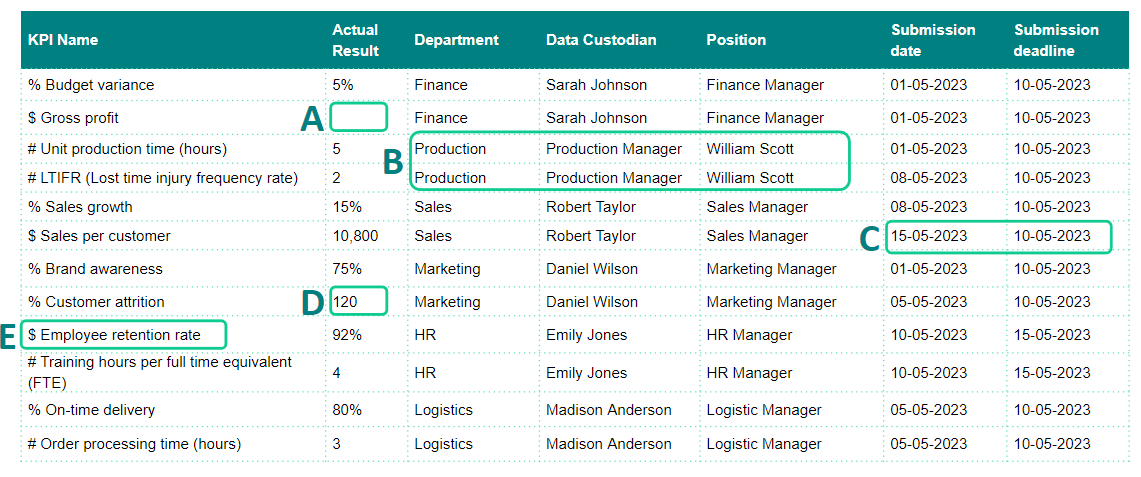
Figure 2. Sample quality troubled dataset | Source: The KPI Institute
A – Completeness: There is a missing value in the Actual Result column. One way to prevent this is to develop and utilize a data collection template that clearly outlines the necessary data fields. It is also important to regularly review the completeness of the data and address missing information that affects analysis.
B – Consistency: The structure of the data does not correspond with the template, the name, and the position of the Data Custodian being switched. To prevent this issue, one must make sure the data presents the same values across different systems and follows the same structure.
Read More >> All About That Data – Sources and Collection Methods
C – Timeliness: This issue pertains to the data being received after the specified deadline. One potential solution is to establish a data collection cycle time and set clear deadlines for data submission. Communicating these deadlines to all relevant parties and sending reminders for data submission can also help address this issue.
D – Conformity: The KPI is expressed as a percentage rate, but the data provided for the result includes a numerical value. To ensure conformity, organizations must provide clear guidelines on data format and how the KPI should be calculated.
E – Accuracy: This issue concerns the usage of an inappropriate sign. The KPI measures a rate, but the sign used in the KPI name is “$.” To ensure accuracy, one should make sure the data reflects real information, including the use of appropriate units. To adhere to accuracy, The KPI Institute developed a naming standard, which designates the symbols ”#” for units, ”%” for rates, and ”$” specifically for monetary value.
Read More >> Why is Data Integration Important and How Can We Achieve It?
Managing Data Quality Dimensions and KPIs
Maintaining data quality is essential to generate meaningful and effective KPIs. Reliable data ensures that business decisions are based on trustworthy information, resulting in improved marketing, increased customer satisfaction, enhanced internal processes, and reduced costs.
On the other hand, unreliable data can cause significant challenges. KPIs based on inaccurate data lead to wrong decisions, resulting in wasted resources and a negative impact on the organization’s performance. Poor data quality can impede the identification of trends or the accuracy of forecasts, leading to missed opportunities. In addition, it can hold back innovation, causing businesses to lose competitiveness.
Therefore, it is recommended that organizations prioritize data quality management and take actions to assess and improve data quality to enhance KPIs and drive business success.
Enhance your understanding of KPIs and read more about them on our KPI section.
**********
Editor’s Note: This article was originally published in Performance Magazine: Issue No. 26, 2023 – Data Analytics Edition and has been updated as of September 17, 2024.