Leveraging Natural Language Processing in the SMB Lending Process
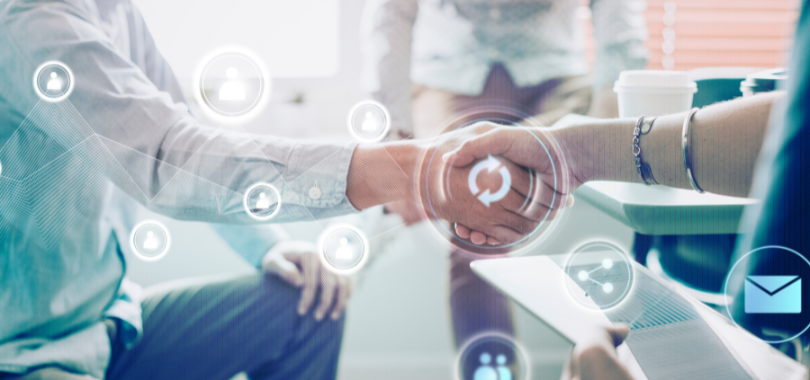
Image Source rawpixel.com via Freepik
Natural language processing (NLP) is a game-changer in small and medium-sized businesses (SMB) lending. SMB lending is widely considered a slow, protracted process that frustrates both borrowers and lenders. Unlike larger corporations, most SMBs are informal businesses with limited financial records. This requires borrowers to submit a plethora of both digital and paper- based legal documents, collateral deeds, financial reports, and even business plans—all of which need to be verified and analyzed meticulously to determine the lender’s credit worthiness. This makes it difficult for SMBs to access the much-needed financial products in a timely manner as the end-to-end loan process would take on average between three to six weeks to close.
To be profitable, the sector must also rely on the volume of borrowers while also adhering to tight regulations set by the central bank. This effort takes a lot of resources, especially manpower. With the addition of risk due to fraud and loan defaults, there is a need for a system that assists lenders simplifying such tedious processes while also maintaining overall credit quality, measured by KPIs such as % Loan delinquency and % Bank non performing loan and gross loan.
Enter NLP, a form of artificial intelligence (AI) that allows computers to understand both spoken and written human language. NLP has a lot of potential due to its ability to automatically “read” and extract useful information from both structured data (ie. sales reports) and unstructured data (ie. social media data). According to a survey in 2023, NLP has been widely used for data recognition and extraction, human intention classification (ie.g. chatbots) and natural language generation (ie.g. ChatGPT). These solutions utilizations offer organizations increased in cost efficiency through improvements in key performance indicators (KPIs) such as % Process efficiency and # Process completion time.
Read More: The New Wave: How Bank Relationship Managers Embrace Technology to Build Trust
How NLP Can Optimize SMB Lending Efficiency
NLP has the potential to increase a bank’s business volume by increasing the number of loan applications and decreasing the time it takes to process each applications—measured using KPIs such as # New loan inquiries, # Process completion time, and % Process efficiency ratio. Its main role in the SMB lending process is automating menial tasks, including data extraction from many paper-based documents.
Data points such as business history, revenue, expenses, and liabilities can be simultaneously extracted and validated from borrowers’ identification, bank statement, financial report, and business plans, reducing the effort it takes to go through these documents manually. These improvements can be measured using KPIs such as # Report processing time. In addition, NLP can also help streamline compliance and legal review processes as it can scan legal documents to ensure that they comply with regulatory requirements or identify any documents that need to be reassessed by lending officers.
NLP’s ability to “read” and analyze unstructured data can also enhance the credit risk assessment process. Information from news articles, social media posts, and financial news can be an additional layer of analysis that provides novel insights that traditional financial metrics might miss, such as economic and financial sentiment. Data-driven pricing analysis can also ensure that lenders recommend the most competitive interest rates to potential borrowers.
NLP can also help integrate data from various sources, including emails, voice transcripts, and other communication channels, providing a comprehensive view of the applicant’s profile and history. This allows lending officers to focus on the analysis and information selection that would help credit approval, which can lead to improved scores in the # Loan officer productivity indicator.
Perhaps more exciting is the future development of AI from companies such as Thelightbulb, which would allow AI to “read minds” by collecting and analyzing unconscious, non-verbal responses, and other biometric cues. This would assist lending officers in analyzing the behavior of a potential borrower’s characteristics during interviews and knowing their customer’s process, which enhances credit risk assessment and helps lenders understand their customers’ needs.
Read More: Is the Pay-for-Performance Scheme in the Public Sector Effective?
Integrating NLP into SMB Lending Practices
Despite the numerous benefits of using NLP in the SMB lending process, the inherent risk of lending and the tight regulation mixed with the current capability of AI tools remind us that the role of humans remains very important in selecting and analyzing the right set of data. To ensure effective and cost-effective adoption of the technology, financial institutions must fully understand the specific part of the process they wish to enhance. This will involve implementing measurement tools and indicators that can quantify the amount of improvement the technology can bring to the table. Talents must also receive training, not only to operate the technology, but also to understand the boundaries between their expertise and AI capabilities. Furthermore, the company must also ensure that it already has an internal AI governance framework and regular audit systems in place to establish accountability and fairness in the use of technology.
Click here for more in-depth articles and interviews that discuss how artificial intelligence can be integrated into strategy.
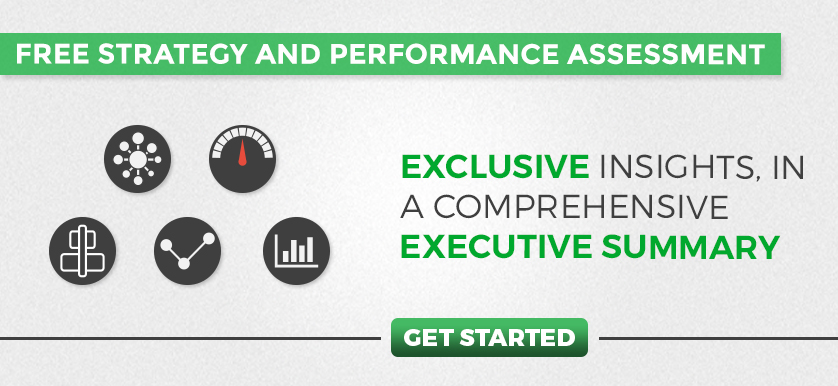
Tags: artificial intelligence, Financial Sector, Natural Language Processing, NLP, SMB lending