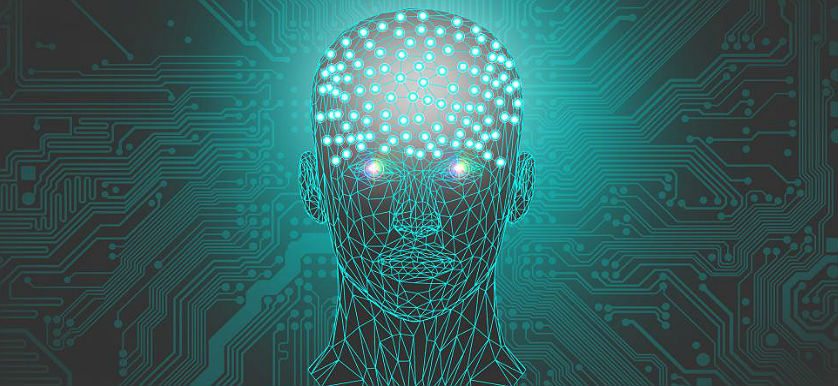
Artificial intelligence (AI) is becoming an increasingly prominent aspect of the contemporary technology landscape. Utilizing the latest development in the AI sphere can definitely give a company an advantage over its competitors. But in order to achieve this, it’s important to understand some of the key concepts involved within this technology.
Central to the whole notion of AI is learning. Ultimately, artificial intelligence represents the attempt to teach computers to learn; something that has been impossible historically. And when trying to understand modern AI, it is vital to comprehend precisely what is meant by the terms ‘deep learning’ and ‘machine learning’, and the differences between the two.
Machine Learning
Machine learning is what the uninitiated would typically associate with AI, being based around using statistical techniques to help computers to learn. Machine learning has been defined as “algorithms that parse data, learn from that data, and then apply what they’ve learned to make informed decisions”.
This key technique in artificial intelligence has become widely used across multiple industries. It is perhaps most commonly used in the field of generating personal recommendations for people on the Internet. Complex algorithms are used in order to generate user profiles, which are then matched with suitable information by the computer in question.
Deep Learning
Deep learning is technically a sub-field of machine learning, with a specific remit. Deep learning has the perhaps more ambitious aim of attempting to teach computers to carry out tasks that come relatively naturally to human beings. This is an aspect of artificial intelligence that is expected to grow in the coming years, as the technology involved becomes more sophisticated.
One of the most high-profile uses of deep learning has been in the field of self-driving cars. Already, companies such as Tesla have produced technology which enables vehicles to effectively pilot themselves, even if some human involvement is still legally requisite. Deep learning involvement in this field is expected to amplify, as people become more accustomed to self-driving cars as a concept.
Let’s compare them
Perhaps the most obvious fundamental difference between machine learning and deep learning is that the former requires some guidance. While machine learning models will become better at the task assigned to them over a period of time, they still require helping hands from humans. Deep learning is intended to master and refine a computer’s ability at a particular task, without any human involvement whatsoever.
As mentioned above, machine learning is based on algorithms, which it then uses to parse data and makes decisions on the basis of this learning process. Whereas deep learning structure algorithms in layers, in order to create what is referred to as an ‘artificial neural network’. Once this structure is created, it can effectively learn on its own.
The performance of deep learning programs produced by Google in the fields of chess and Go have demonstrated the possibilities of this technology. Meanwhile, the technology is also expected to play a major role in multiple industries in the coming years, with finance and healthcare both at the top of the list. As the field continues to develop, AI certainly offers many exciting opportunities for businesses.
Image source: